Deciphering FDA's 7-Step Framework For AI-Driven Decision-Making
By Fahimeh Mirakhori, M.Sc., Ph.D.
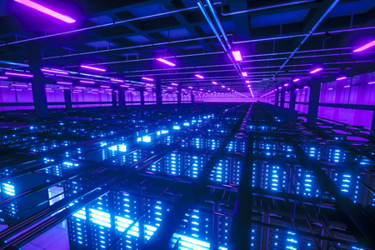
AI is rapidly transforming industries, especially in healthcare and life sciences, offering new possibilities in drug development.1-3 As AI evolves, regulatory agencies are working to integrate it into their oversight processes, ensuring it is both effective and safe.4-8 The U.S. FDA has taken a pioneering step with its January draft guidance on AI-driven regulatory decision-making for drug and biological products.6 This guidance marks a critical effort to address the challenges of using AI in drug development and to establish a framework for regulatory decisions that incorporate the evolving capabilities of AI.
A key component of the FDA’s guidance is the 7-step framework for assessing AI model credibility, which aims to ensure that AI systems used in drug development are robust, reliable, and aligned with regulatory expectations. This structured, risk-based approach considers the intended context of use (COU) and the associated risks, helping regulators and developers determine whether AI models can be trusted to support critical decisions in drug development. The framework focuses on defining the regulatory question, evaluating model risk, and verifying model reliability through a series of comprehensive steps.5,6 Through this framework, the FDA clarifies how AI models should be evaluated across their life cycle, ensuring that AI-driven innovations meet regulatory standards while maintaining transparency. As part of this process, the FDA encourages early engagement with stakeholders to refine the assessment activities and ensure models are continuously updated to align with changing performance and regulatory requirements.
The U.S. FDA’s 7-Step, Risk-Based Framework for Assessing AI Model Credibility in Drug and Biological Product Development Regulatory Decision-Making
The FDA’s draft guidance introduces a 7-step framework to ensure AI models used in drug development are robust, reliable, and meet regulatory standards. This framework evaluates AI models based on their COU and associated risks, emphasizing key aspects like model definition, risk measurement, analysis and control, and similar quality management systems.6,9,10
Data quality and model generalizability are crucial for making informed regulatory decisions in drug development and clinical trials. While AI’s adaptive nature supports dynamic data quality assurance (QA) metrics improvements, continuous oversight is crucial to validate AI models, mitigate errors, and ensure robustness during updates and real-world applications. Acceptance testing, which ensures AI systems are validated before deployment, is essential for verifying that training and testing datasets are complete, accurate, representative, and unbiased. Generalizability metrics like cross-validation, external validation, and sensitivity analysis ensure models perform effectively across diverse real-world settings. Bayesian models are particularly effective in addressing data quality and generalizability challenges. These models excel in uncertainty estimation, model validation, and built-in QC/QA mechanisms, making them foundational in AI/ML algorithms, especially when working with smaller datasets or uncertain data.11-14
Integrating new digital technologies, such as real-world evidence (RWE) and real-world data (RWD), enhances the AI model’s performance and ensures its adaptability over time, where interoperability difficulties, irregular EHR coverage, and data quality inconsistencies might limit reliable analysis.11 Among other AI-driven predictive statistical tools, Bayesian methods allow AI models to refine iteratively in adaptive trials. This iterative process improves decision-making by incorporating new data. These models improve adaptability and decision-making, particularly when traditional trials aren’t feasible while refining predictions and strengthening AI-driven evaluations of drugs and devices.12-14
Click for full size.
Step 1 — Define Phase
The first step in the framework is to define the specific regulatory question the AI model will address, considering the regulatory context, intended outcome, and supporting evidence. For example, in clinical development, if Drug A is associated with a life-threatening adverse reaction, the regulatory question might be: “Which participants are low-risk and do not need inpatient monitoring?” This focuses on patient safety and monitoring decisions, with evidence from prior trials.
In manufacturing, for Drug B, a critical quality attribute is fill volume. An AI-based system is proposed to assess vial fill levels. The regulatory question here could be: “Do vials of Drug B meet fill volume specifications?” This ensures product quality and regulatory compliance, supported by historical manufacturing data and the AI model’s evaluation capabilities.
In both cases, defining the regulatory question ensures the AI model supports decisions that improve safety, quality, and compliance. The model’s effectiveness must be backed by evidence to meet regulatory standards for decision-making and product approval.
Step 2 — Define And Measure Phases
The next step is defining the AI model’s COU, including its role, scope, and how its outputs will address the regulatory question. The model’s inputs, outputs, and integration with other data sources should also be clearly defined. In clinical development, for example, the AI model predicts a participant’s risk for a life-threatening adverse reaction to Drug A based on baseline characteristics and lab values. The model will stratify participants into low- and high-risk groups, helping determine the need for inpatient or outpatient monitoring, but clinical decision-making will remain primary. In manufacturing, the AI model analyzes vial images to detect deviations in fill volume. However, the model’s findings will complement, not replace, existing quality control measures, like batch-level volume verification, to ensure thorough product quality assessment.
This step must also address data quality — defining criteria for completeness, accuracy, consistency, and representativeness. Clear guidelines for ongoing data validation should be provided, particularly when RWE is used in AI models. Effective data validation processes ensure the model remains reliable and adaptable to new data, supporting high performance and regulatory compliance throughout the AI life cycle.
Step 3 — Analyze Phase To Assess Model Risks
Assessing the risk of an AI model is critical. Model risk is determined by two factors:
- Model Influence measures the importance of the model’s output in decision-making. The AI model may solely determine participant monitoring in clinical development, making its influence high. The model’s output complements existing quality control in manufacturing, making its influence medium or low.
- Decision Consequence reflects the outcomes of incorrect decisions. In clinical development, incorrect risk stratification can lead to life-threatening adverse events. In manufacturing, incorrect fill volume can result in medication errors or dosage issues, leading to high consequences.
To classify model risk, sponsors should evaluate error severity, the probability of adverse outcomes, and detectability using tools like risk matrices. They should also assess whether the AI model uses data from various sources (e.g., clinical trials, RWD) and ensure robustness against threats like cybersecurity risks.
For example, in clinical development, high model influence and high decision consequences lead to a high-risk classification. While the decision consequence is high in manufacturing, the reduced model influence due to complementary quality control results in medium risk.
Incorporating QA metrics to address data quality (accuracy, completeness, consistency) is essential. Establishing bias detection and error tolerance ensures that the model performs within acceptable limits. Validating data sources (e.g., clinical trials, insurance claims) and ensuring well-annotated, unbiased data improve model generalizability and confidence in its reliability for regulatory use. This process ensures AI innovations meet regulatory standards while safeguarding patient safety. This risk assessment mirrors the failure mode and effects analysis (FMEA), where risks are prioritized based on severity, probability, and detectability, with both frameworks requiring continuous monitoring to maintain effectiveness.
Step 4 — Improve Phase
This step involves developing a credibility assessment plan to establish the AI model’s reliability, once model risk and COU are defined. The plan should include criteria for evaluating the model’s accuracy, reliability, robustness, and methods for mitigating biases. High-risk models require detailed validation and documentation, while low-risk models need minimal assessments. The plan should define key validation metrics (e.g., performance indicators, quality control) and include bias mitigation protocols. Risk-specific strategies should be tailored to the model’s impact on decision-making, with more detailed strategies for high-risk models.
The plan should outline how and when the sponsor will seek FDA feedback, with early discussions to refine the plan and meet regulatory expectations. This engagement ensures alignment with regulatory standards and helps address challenges. The FDA encourages early engagement, particularly for high-risk models. As AI evolves, assessments should be updated to reflect changing performance.
Advanced QA techniques like autonomous test generation, self-healing mechanisms, and cognitive test exploration can enhance the assessment process. Incorporating external control arms (ECAs) and adaptive trial designs is helpful for situations where traditional methods are limited, such as small patient populations or rare diseases. ECAs use external data sources (e.g., previous trials, observational studies, RWE) to improve model accuracy.
Ongoing monitoring and model recalibration post-approval ensure the AI model’s effectiveness as new data is integrated, maintaining regulatory alignment. By adopting these practices, sponsors can ensure their AI models are robust, reliable, and meet regulatory standards, building trust in AI-driven drug development.
Step 5 — Improve Phase: Execute The Plan
After developing the credibility assessment plan, the next step is to execute it. This involves implementing the planned activities such as testing, validation, and error mitigations to establish the credibility of the AI model. Throughout this phase, sponsors should consult with the FDA to address any challenges, refine the credibility assessment activities, and ensure that expectations regarding the credibility assessment are met based on model risk and COU. Early discussions with the FDA can help identify potential challenges and determine effective strategies for addressing them.
Document all execution details, including the results, deviations, and performance metrics of the model. These records will provide insights into the model’s effectiveness and reliability, ensuring it performs as intended within its regulatory context. The execution phase plays a crucial role in ensuring that the AI model is rigorously tested and evaluated, laying the foundation for its effectiveness in regulatory decision-making and future applications.
Detailed QA procedures should also be followed to maintain consistency and minimize errors, including reviewing test results for discrepancies and performing routine checks for data integrity and traceability. Additionally, implementing robust monitoring systems during the execution phase will help track performance over time and ensure that the AI model adapts effectively to new data without introducing biases or errors. The execution phase is pivotal in ensuring that the AI model is rigorously tested and evaluated, laying the foundation for its effectiveness in regulatory decision-making and future applications.
Step 6 — Control Phase: Document The Results
The credibility assessment report compiles the findings and activities from the credibility assessment plan, highlighting any deviations and providing evidence of the AI model’s suitability for its COU. This report is essential for demonstrating compliance and ensuring the model meets its intended purpose. Sponsors should engage with the FDA early (Step 4) to determine the report’s format and timing. It may be submitted as part of a regulatory submission, included in a meeting package, or held for inspection, to be provided upon request. The report is crucial for ensuring transparency and regulatory alignment.
The report must undergo rigorous QA to check for discrepancies, validate test results, and ensure data integrity. QA reviews should ensure the report meets regulatory standards, is inspection-ready, and that all data changes are properly traced.
Step 7 — Control And Continuous Improvement Phase: Determine Model Adequacy For COU
The final step is to evaluate whether the AI model meets predefined credibility standards for its COU. Based on the credibility assessment report, the model’s suitability for the defined COU will be determined. If the credibility is found inadequate for the associated risk level, several options are available to address this:
- Incorporate additional evidence to strengthen the model’s credibility.
- Increase the rigor of the assessment by adding more development data or refining the validation methods.
- Adjust the COU to better align with the model’s capabilities.
- Revise the model iteratively to meet the required standards.
If no adjustments can adequately establish the model’s credibility, it may need to be excluded or revised. This step ensures the AI model meets the necessary standards for its specific regulatory application.
Additionally, sponsors should outline life cycle maintenance strategies, including ongoing monitoring, updates, and continuous performance validation, to ensure the model remains reliable and aligned with regulatory expectations over time.
Conclusion
The FDA’s 7-step credibility framework plays a central role in assessing AI model reliability, ensuring models meet predefined standards for accuracy, robustness, and transparency. Enhancing the framework with strategies like adaptive trial designs, ECAs, and continuous oversight can further strengthen regulatory decision-making processes. The integration of RWD throughout the drug life cycle, including post-market surveillance, is crucial to building trust in AI models. By including patient perspectives and aligning with global standards, the FDA can ensure that AI applications remain ethical, equitable, and patient-centric.
We emphasize refining the 7-step framework by incorporating tools like Bayesian and RWE to keep pace with AI advancements in drug development. Continuous stakeholder feedback and updates to the guidance are crucial to creating a regulatory framework that balances innovation with safety, ensuring AI models are effective and equitable in healthcare and life sciences decision-making.
References:
- Mirakhori, F. and S.K. Niazi, Harnessing the AI/ML in Drug and Biological Products Discovery and Development: The Regulatory Perspective. Pharmaceuticals, 2025. 18(1): p. 47
- Chawla, M., Artificial Intelligence in Drug Discovery: Transforming the Future of Medicine. Premier Journal of Science, 2024.
- Bose, A., Regulatory initiatives for artificial intelligence applications: Regulatory writing implications. Medical Writing, 2023.
- Hooshidary, S. Artificial Intelligence in Government: The Federal and State Landscape. 2024.
- HHS, U.G. AI-Focussed Policies. 2024.
- FDA, U. Considerations for the Use of Artificial Intelligence to Support Regulatory Decision-Making for Drug and Biological Products. Guidance for Industry and Other Interested Parties DRAFT GUIDANCEs. 2025.
- Agency, E.M. Artificial intelligence workplan to guide use of AI in medicines regulation. 2023.
- EMA. White Paper on Artificial Intelligence: a European approach to excellence and trust. 2020 [cited 2025 Jan 11th]; Available from: https://commission.europa.eu/document/d2ec4039-c5be-423a-81ef-b9e44e79825b_en.
- Ogrizović, M., D. Drašković, and D. Bojić, Quality assurance strategies for machine learning applications in big data analytics: an overview. Journal of Big Data, 2024. 11(1): p. 156.
- Mahmood, U., et al., Artificial intelligence in medicine: mitigating risks and maximizing benefits via quality assurance, quality control, and acceptance testing. BJR|Artificial Intelligence, 2024. 1(1).
- Li, M., et al., Integrating Real-World Evidence in the Regulatory Decision-Making Process: A Systematic Analysis of Experiences in the US, EU, and China Using a Logic Model. Frontiers in Medicine, 2021. 8.
- FDA, U. Guidance for the Use of Bayesian Statistics in Medical Device Clinical Trials. 2010. [cited 2025 Feb.1th]; Available from: https://www.fda.gov/regulatory-information/search-fda-guidance-documents/guidance-use-bayesian-statistics-medical-device-clinical-trials.
- Kidwell, K.M., et al., Application of Bayesian methods to accelerate rare disease drug development: scopes and hurdles. Orphanet Journal of Rare Diseases, 2022. 17(1): p. 186.
- FDA, U. Quality Systems Approach to Pharmaceutical Current Good Manufacturing Practice Regulations. 2006 [cited 2025 Jan 11th]; Available from: https://www.fda.gov/regulatory-information/search-fda-guidance-documents/quality-systems-approach-pharmaceutical-current-good-manufacturing-practice-regulations.
About The Author:
Fahimeh Mirakhori, M.Sc., Ph.D. is a consultant who addresses scientific, technical, and regulatory challenges in cell and gene therapy, genome editing, regenerative medicine, and biologics product development. Her areas of expertise include autologous and allogeneic engineered cell therapeutics (CAR-T, CAR-NK, iPSCs), viral vectors (AAV, LVV), regulatory CMC, as well as process and analytical development. She earned her Ph.D. from the University of Tehran and completed her postdoctoral fellowship at Johns Hopkins University School of Medicine. She has held diverse roles in the industry, including at AstraZeneca, acquiring broad experience across various biotechnology modalities. She is also an adjunct professor at the University of Maryland.